Quant Trading: What is it? Who does it? What are the challenges?
Quant Trading: What is it? Who does it? What are the challenges? (12 min read)
The idea of this article is to provide an overview of what quantitative trading is, what trading styles use it, what types of groups use it, the types of roles available with quantitative trading and where it is all heading.
What is Quant Trading?
Quantitative trading, or quant trading, is a trading strategy that uses mathematical and statistical models to analyse financial data and make investment decisions. It involves using algorithms and computer programs to identify patterns and trends in market data and execute trades based on those patterns.
Quantitative traders use large data sets to develop and test their trading algorithms. They often rely on high-speed computers and other advanced technologies to execute trades quickly and efficiently. They may also use a variety of financial instruments, including stocks, options, futures, currencies, and digital assets, to build and manage their portfolios.
Hedge funds, investment banks, and other institutional investors use quantitative trading. Still, it is also increasingly being used by individual traders and investors with access to sophisticated trading platforms and tools. Quant trading aims to generate consistent profits over time by identifying and exploiting market inefficiencies and anomalies.
Quant Trading History
Quantitative trading has grown significantly in usage over the last 50 years. In the 1970s, quantitative trading was in its infancy, and most trading was done manually using fundamental analysis and technical analysis. However, the introduction of computers and the development of quantitative models in the 1980s led to an explosion in quantitative trading strategies.
In the 1990s, the rise of high-frequency trading and the development of algorithmic trading systems further accelerated the adoption of quantitative trading. By the early 2000s, quantitative trading had become dominant in financial markets, accounting for a significant portion of trading volume in many asset classes. It has only grown since.
"According to data compiled by Goldman Sachs and shared by Analysing Alpha, about 60%-70% of trading in equities in 2016 was via algorithmic trading, while about 40%-50% of futures trading was contributed by algorithmic trading. About 35%-50% of the commodity trading volume is generated by algorithmic trading, and similarly, nearly 40% of options trading was via trading algorithms. During that period, Forex recorded about 20%-30% of algorithmic trading, while fixed-income trading had about 10% of algorithmic trading. see the chart below:"[1][2]
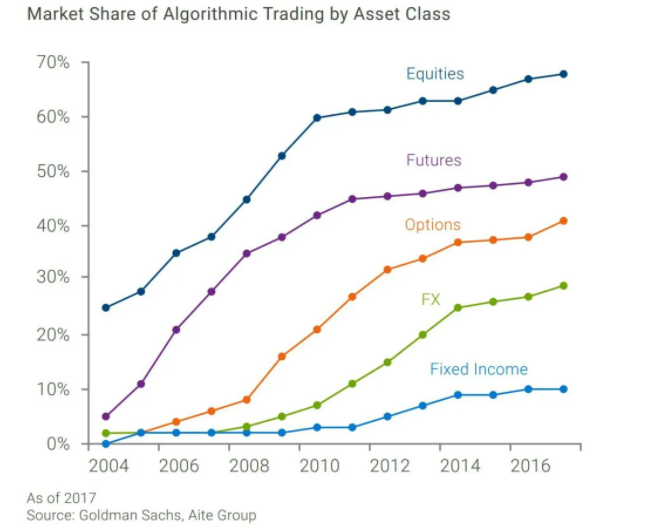
Overall, the increasing availability of data, advances in technology and computing power, and the development of sophisticated quantitative models and algorithms have fuelled the growth of quantitative trading over the last 50 years.
Example Quant Trading Strategies
Here are some examples of quantitative trading strategies:
- Statistical arbitrage: This strategy involves identifying mispricings in securities that highly correlate with each other and then profiting from the price differences. Statistical arbitrage often consists of high-frequency trading and requires sophisticated statistical models to identify the mispricings. It also comes in the form of pairs trading. Some argue they are the same, while others feel they have inherent differences.
- Momentum trading: This strategy involves buying securities that have shown strong price momentum in the past and selling those that have demonstrated weak momentum. Momentum traders may use technical analysis and machine learning techniques to identify trends and patterns in market data. Also known as trend following - but again, some will argue trend is slightly different from momentum! Momentum tends to focus on breakouts. In contrast, trend / CTA strategies are looking at longer-term tailwinds.
- Mean reversion trading: This strategy involves buying undervalued securities and selling those overvalued, expecting prices to revert to their long-term averages eventually. Mean reversion traders may use statistical models and other quantitative techniques to identify undervalued and overvalued securities. Some argue mean reversion and stat-arb are the same things, which they can be, but they can look at things differently, so some will argue MR is a form of stat-arb but not all that stat-arb is pure MR. Pairs trading could also be classified as MR for some and not for others.
- High-frequency trading: This strategy uses sophisticated algorithms and high-speed computers to execute trades quickly and efficiently. High-frequency traders often use quantitative models to identify market data patterns and make trades based on those patterns. HFT could be market-making, stat-arb or some other form of arbitrage. The key characteristics are its speed and its prediction engine for short-price prediction.
- Multi-factor trading: Multi-factor trading is quantitative trading that involves using multiple factors or variables to determine trading decisions. It aims to reduce risk and improve returns by capturing a broader range of information. It incorporates fundamental, technical, and macroeconomic factors.
These are just a few examples of the many quantitative trading strategies. The specific approach used by quantitative traders will depend on their investment objectives, risk tolerance, and the markets they are trading. It will likely be a blend of these mentioned. Some will blend two or three of the above or build new strategies based on that market - such as index arbitrage, global macro with RV & Carry types strategies etc.
Critical features of quant trading
Here are some of the critical features of quant trading:
- Data-driven: Relies on data, statistical analysis, and machine learning algorithms.
- Objective: Removes subjective biases from decision-making.
- Systematic: Adheres to defined rules and procedures for trades.
- Automation: Employs automated trading systems for quick, efficient executions.
- Risk management: Utilises rigorous techniques to limit losses.
- Medium to High frequency: Involves large numbers of trades in short time frames with high-speed computers and algorithms.
Who does quant trading?
Various financial institutions use quantitative trading, including hedge funds, investment banks, asset management firms, and proprietary trading firms.
Hedge funds are some of the most significant users of quantitative trading strategies. Many of the most successful hedge funds in the world, such as Renaissance Technologies, Two Sigma Investments, and D. E. Shaw & Co., rely heavily on quantitative models and algorithms to make investment decisions. Their quant trading techniques differ based on investment philosophy, time horizons, and strategies.
Asset managers utilise quantitative trading across many areas. Some may use fully systematic trading strategies, while others use them more strictly for portfolio construction, optimisation, and risk management.
Prop trading houses have become increasingly quantitative in their approach. Some of the most famous prop trading shops, such as Tower Research and Jump Trading, focus predominantly on HFT trading, which would be impossible without quantitative-based trading.
Across all three hedge funds, prop shops and asset managers, different setups and styles influence the quantitative roles and strategies. While imperfect, you can divide groups into a pod or research setup. Typically the pod structure is found in multi-strategy groups with teams of portfolio managers. In contrast, the research-style shops have more singularly focused funds, where they run a large fund with multiple researchers adding value.
Investment banks use quant trading for algorithmic execution, electronic market making, and central risk trading strategies across various financial instruments.
Individual investors engage in quantitative trading through platforms and tools that offer data analysis, algorithms, and resources for data-driven decisions. This area is rising as data becomes more available and cheaper, such as with crypto exchanges providing their price feed data freely, lowering the entry bar. Websites like QuantConnect allow individuals to backtest strategies better. Some hedge funds have been capturing more of these people by allowing external contributors to provide signals, such as Qube Research & Technologies.[3]
Types of Roles at Hedge Funds
Quantitative trading involves a range of jobs that require different skills and expertise.
Quantitative Portfolio Manager: A Quant PM uses quantitative models and algorithms to manage investment portfolios. They are responsible for developing and executing trading strategies to generate profits for clients or their firm's accounts. A quant portfolio manager typically works closely with quantitative researchers to develop and refine trading models and algorithms. They may work alongside other professionals, such as data analysts, software developers, traders, and risk managers, to develop and execute trading strategies.
The role of a quant portfolio manager focuses on investment decision-making and portfolio management; depending on the size of the team, they may also focus on researching, developing, and implementing quantitative models and signals. They must understand quantitative techniques and communicate and explain complex models and investment strategies to clients and stakeholders. A quant portfolio manager is typically a more senior position that requires extensive experience and expertise in quantitative research, financial modelling, and investment management.
Quantitative Researchers or Quant Analysts (quants):
Quantitative researchers and quantitative analysts are professionals in quantitative finance, but they have different roles and responsibilities.
A quant researcher is responsible for developing new models and trading strategies by conducting research and analysing financial data, aiming to either generate alpha through an automated strategy or improve the risk management & execution of the automated strategy. They work on developing mathematical models and statistical algorithms to identify patterns in data and predict future market movements.
On the other hand, a quant analyst is responsible for researching and modelling securities. They analyse data, monitor trading systems, and identify potential market opportunities or risks. Quant analysts work closely with traders to help them make informed decisions based on the models and data analysis.
Overall, while both roles require strong analytical skills and a background in mathematics, quant researchers are more focused on models and strategies for systematic trading. In contrast, quant analyst models return profiles, yield curves, pricing of securities and more to provide alpha generation or risk management ideas to the portfolio manager, who trades in a non-automated manner.
Data Scientists or Data Analysts:
Data scientists at hedge funds develop and implement trading strategies by analysing large volumes of financial data. They handle data analysis, model development, testing, evaluation, and data management, contributing to data-driven trading strategies that generate alpha and improve risk management.
Data analysts gather and interpret data to inform business decisions. They use statistical techniques and visualisation tools to identify trends and patterns, focusing on descriptive analytics for insight and decision-making.
Data scientists, conversely, have a broader focus on developing data-driven models and algorithms to tackle complex problems. They possess strong mathematics, statistics, and computer science backgrounds, employing advanced techniques like machine learning and artificial intelligence for predictive and prescriptive analytics. Typically holding a PhD, data scientists apply advanced ML techniques like deep learning to create signals, setting them apart from data analysts.
Software Developers: Software developers build and maintain software and algorithms for quantitative trading. They may develop trading platforms, backtesting tools, and other supporting software. Specialisation in a programming language, such as C++, Java, or Python, is required. While in-depth financial knowledge is not essential, expertise in a specific market area, like market microstructure, is highly valued.
Traders: Traders execute trades using signals from quantitative models and algorithms, monitor markets, and adjust strategies in real time. Roles may vary, with some being execution traders or relying on algorithms. Top groups often have in-house algo execution quants.
Risk Managers: Risk managers monitor and manage risks linked to quantitative trading strategies. They use diversification, hedging, and stop-loss orders and have finance, economics, or risk management degrees. They are skilled in quantitative analysis, financial modelling, and regulatory compliance.
Quant trading involves a range of jobs that require a combination of skills in mathematics, computer science, finance, and business. Each job's roles and responsibilities depend on the institution and the trading strategy.
Potential Issues for Building a Quant Trading Business
Hedge funds face several challenges when building a quant trading business, including:
- Data quality & availability: High-quality data requires investment in data infrastructure and analytics tools.
- Talent acquisition & retention: Attracting specialised talent (PMs, data scientists, software engineers, quantitative analysts) is complex due to high demand and competition. Offering competitive compensation, professional development, and work-life balance is critical.
- Technology and infrastructure: Quantitative trading needs sophisticated technology infrastructure for efficient trade execution. Hedge funds must invest in the right technology to execute trades quickly and efficiently.
- Regulatory compliance: Hedge funds must comply with various regulations, including reporting requirements, risk management, and compliance with securities laws. This changed recently, with the SEC requiring big funds to report losses.[4]
- Risk management: Robust systems are needed to manage market, credit, and operational risks.
- Performance measurement & evaluation: Developing benchmarks and metrics helps measure quantitative trading strategies' performance and attract investors and employees.
- Raising capital: Securing adequate funding from investors is essential to support the growth and operations of a quant trading business, which may require showcasing strong performance, risk management, and a competitive edge in the market.
- Successfully building a quant trading business requires considerable talent, technology, infrastructure, and risk management investment to create effective strategies and generate returns for investors.
The Future of Quant Trading: Key Trends and Challenges
The future of quant trading is likely to be shaped by a range of technological, economic, and regulatory factors. Some potential trends and developments in quant trading include AI, data, new assets, and more. As we explore these trends, we must recognise how they will impact the industry and how quant traders operate. I wrote about the Future of Quant Trading here.
Ai & ML - Machine learning and artificial intelligence have been widely used in quant trading for many years and will only increase. Advances in these technologies are expected to lead to more sophisticated and adaptive trading models and more efficient and effective data analysis. This increase in technology may revolutionise how quant trading is approached and help traders gain a competitive edge in the market. I discussed it more here, Ai and Machine Learning in Quant Trading.[5]
It is just the beginning of the journey. Many firms were looking at Ai and ML in their trading strategies, how they analyse this data set, and how they make better predictions. However, the release of ChatGPT and OpenAi has flipped this. It is no longer only about how to incorporate Ai into the tip of the spear work, the alpha strategies. But how can we use Ai across our whole business? Ai for marketing to better raise capital, Ai for coders to increase their efficiency by 50% or more. Ai to ask finance-related questions by researchers or a CFO etc., with BloombergGPT.[6] Citadel is already looking at bringing it into its business.[7]
Alt Data - Quant traders are turning to alternative data sources as traditional data sources become saturated. These alternative data sources, such as satellite imagery, social media, and internet search data, can provide unique insights into market trends and consumer behaviour. By leveraging these new data sources, traders can identify opportunities and potential risks that may not be evident from traditional data sources alone.
New Assets - Quantitative trading is expanding into new asset classes and markets, such as cryptocurrencies, commodities, and emerging markets, as traders seek to diversify their portfolios and identify new sources of alpha. The growth of these new markets may lead to new trading opportunities and challenges. As the industry evolves, algorithmic trading will take up an increasingly larger share of total trading volume.
ESG - There is a growing focus on sustainability and ESG factors, which will likely impact how quant traders develop trading strategies. There may be an increased demand for strategies incorporating ESG factors and promoting sustainability, aligning with investors' values and interests. As quant trading becomes more popular and mainstream, it is essential for traders to consider the broader implications of their strategies on the environment and society.
Quantum computing - could significantly impact the field of quant trading. The increased processing power could be used to develop more complex and sophisticated trading algorithms and analyse larger and more complex datasets. [8]Quantum Computing for Finance (QCF) is a collaboration between the University of Oxford, JP Morgan, and IBM that aims to develop quantum algorithms for finance. The project is focused on applications such as portfolio optimisation, option pricing, and risk management. However, many technical and practical challenges must be addressed before quantum computing can be widely used in quant trading.
Conclusion
In conclusion, the future of quant trading is likely to be characterised by continued innovation and the adoption of new technologies and data sources. However, quant traders must also navigate a complex and rapidly changing regulatory environment and increased competition from other market participants. Staying ahead in this ever-changing field requires continuous learning about the latest technologies, machine learning advancements, data sources, and more. By doing so, quant traders can stay ahead of the competition and the alpha decay they face, ensuring ongoing success in the industry.
The best way for someone to succeed within quant trading would always be to continue learning in this ever-changing field. Those that stop learning thinking they have a winning strategy, are quickly caught and passed. One must stay in touch with the latest technologies, ML advancements, data sources and more to stay ahead of the competition and the alpha decay they face.
Please let me know what your thoughts are.
Do you think stat-arb and mean reversion are the same or different?
Where do you think Ai will go in Quant Trading?
Please like and comment below.
Thanks for reading, All the Best, Henry.
Founder of QuantLink, a specialist search firm in the quant trading space, aimed at providing unique opportunities and value to our network.
[1] https://www.quantifiedstrategies.com/what-percentage-of-trading-is-algorithmic/#:~:text=In%202021%2C%20nearly%2092%25%20of,the%20time%20of%20the%20report.
[2] https://www.quantifiedstrategies.com/what-percentage-of-trading-is-algorithmic/#:~:text=In%202021%2C%20nearly%2092%25%20of,the%20time%20of%20the%20report.
[3] https://www.qube-rt.com/external-contributors
[4] https://www.bloomberg.com/news/articles/2023-05-03/big-hedge-funds-face-new-72-hour-deadline-to-report-major-losses
[5] https://www.quantlink.co.uk/ai-and-machine-learning-in-quant-trading
[6] https://www.bloomberg.com/company/press/bloomberggpt-50-billion-parameter-llm-tuned-finance/
[7] https://www.bloomberg.com/news/articles/2023-03-07/griffin-says-trying-to-negotiate-enterprise-wide-chatgpt-license
[8] https://www.ibm.com/thought-leadership/institute-business-value/en-us/report/exploring-quantum-financial